Extract unparalleled operational efficiencies
and customer experiences with AI
The challenge of modern networks
The proliferation of connected devices, along with an exponential growth in traffic, has added to the complexity of network and service operations. With new technologies bringing even more devices and services into the network, communications service providers (CSPs) and other network operators are looking to implement new innovations and tools to modernize their operational systems and processes.
Understanding AI-native systems
A new generation of AI-native systems—inherently designed to use AI algorithms and techniques—has emerged to address these challenges and enable intelligent automation.
The following key capabilities are critical because they represent a fundamental shift from traditional systems that use AI as an add-on feature to systems with intelligence and adaptability built into their foundation. In addition to enabling more sophisticated and effective intelligent automation, these systems deliver measurable business outcomes across the entire network service operations lifecycle.
Key capabilities of AI-native systems
Data-driven intelligence: AI-native systems use large volumes of data to learn, reason, and make informed decisions, extracting meaningful insights and patterns.
Continuous learning and adaptability: These systems continuously learn from data, adapt to new information, and refine their models over time to improve performance and accuracy.
Autonomous and dynamic: AI-native systems can adapt to changing environments and operate independently, making decisions without constant human intervention and dynamically adjusting their behavior.
Data challenge and opportunity
Today’s operators are overwhelmed by telemetry data, including topology information, performance metrics, system logs, and alerts. This issue is further amplified by increasingly dynamic networks, where programmable services have replaced traditionally static configurations. While challenging, this big-data problem also presents a unique opportunity for innovation—one that AI and machine learning (ML) are uniquely suited to help solve.
AI is now a core factor for intelligent automation. From enhancing user interactions to navigating complex end-to-end network management challenges, it is becoming more intrinsic to how CSPs architect their IT and network operations, using techniques often called AIOps.
Data security and integrity are also paramount, and operators express significant concerns about data leaving their secure network environments. They need confidence regarding how their network and customer data is trained on large language models (LLMs) and require private hosting options within their control. Clean and accurate data is needed to accelerate AI adoption, ensuring the correct AI techniques and applications for different use cases.
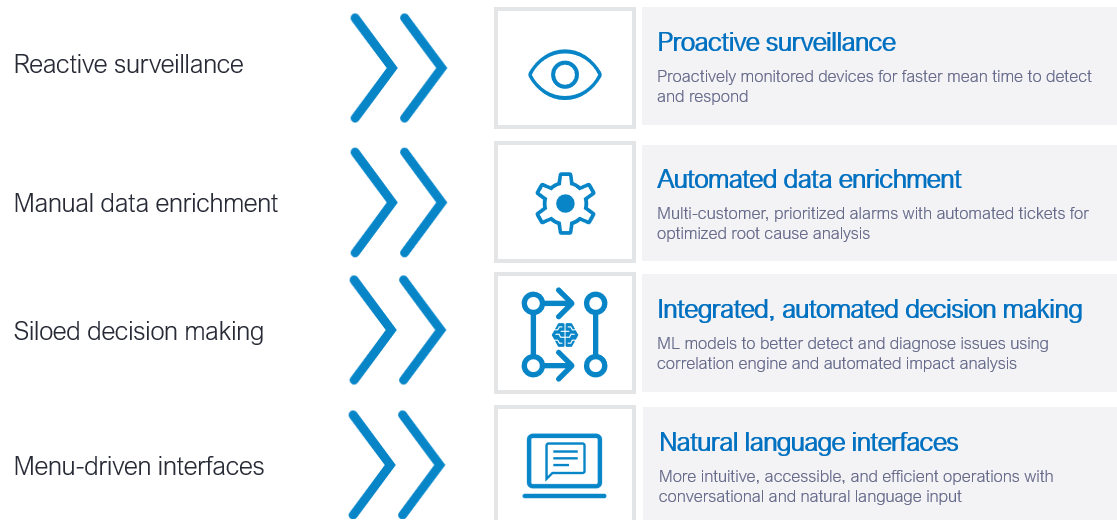
Figure 1. Current method of operations versus the AI-driven method
Operational benefits of AI and analytics
For operators, AI and big data analytics can address these and a wide range of other business and operational needs. For example, AIOps-based continuous learning can help ensure service and network reliability by keeping the network’s health at its optimum. Big data analytics can learn from historical performance metrics and trouble ticketing data to understand normal behavior and predict future network failures. ML models on big data can correlate events, suppress noise, and emphasize high-impact events. Collectively, AIOps, ML, and big data analytics can recommend remediating actions or enable self-optimization and self-healing actions.
Blue Planet’s AI-native approach
The Blue Planet Cloud Native Platform (CNP) and product applications apply AI and big data analytics to historical and real-time data to learn and understand changing network conditions, ensuring network resiliency and service quality of experience. Our AI-native approach is focused on using the right AI for the right use case, while paying particular attention to security and privacy concerns regarding AI and LLMs, and ultimately supporting the transformation to more autonomous, AI-driven network operations.
To learn more about Blue Planet and AI, see Accelerating the shift to AI-driven operations.